Hugging Face Stable Diffusion Models
Artificial intelligence continues to advance at an unprecedented pace, and one of the latest advancements in AI research is the development of Hugging Face stable diffusion models. These models, which are built upon cutting-edge deep learning techniques, have a wide range of applications in various fields such as natural language processing, computer vision, and more. In this article, we will explore the key features and benefits of Hugging Face stable diffusion models and how they can revolutionize AI-driven solutions.
Key Takeaways
– Hugging Face stable diffusion models are state-of-the-art AI models that have gained significant attention in the research community.
– These models leverage deep learning techniques to achieve high performance in various tasks, such as text generation, image recognition, and more.
– Hugging Face models bring advancements in the field of natural language processing, making it easier to generate coherent and contextually relevant text.
**Hugging Face** stable diffusion models are built on a neural architecture called **transformers**, which enable the models to efficiently process and analyze large amounts of data. By using the **self-attention mechanism**, these models can capture long-range dependencies and contextual information, making them highly effective in various AI tasks. Furthermore, Hugging Face models have been pretrained on massive datasets, allowing them to acquire a wide range of knowledge and effectively transfer it to downstream tasks. *This combination of advanced architecture and pretraining makes Hugging Face stable diffusion models powerful tools for AI practitioners.*
Applications of Hugging Face Stable Diffusion Models
Hugging Face stable diffusion models have a wide range of applications across different domains. Here are some notable applications:
- **Text Generation**: Hugging Face models can generate high-quality text in a variety of contexts, including storytelling, chatbots, and content creation. These models can leverage their pretrained knowledge to generate coherent and contextually relevant text.
- **Question Answering**: By understanding the context of a given passage, Hugging Face stable diffusion models can provide accurate answers to specific questions. This is particularly useful in information retrieval systems and chatbot interfaces.
- **Machine Translation**: Hugging Face models excel at language translation tasks by understanding the nuances and context of different languages, enabling accurate and fluent translations.
*With their versatile capabilities, Hugging Face stable diffusion models can enhance a wide range of AI-driven applications and provide more sophisticated and accurate solutions across multiple domains.*
Data Points and Model Comparison
Model | Training Dataset | Accuracy |
---|---|---|
Model A | Large-scale text corpora | 90% |
Model B | General knowledge and language datasets | 87% |
Model C | Custom dataset for specific industry domain | 92% |
Table 1: Accuracy comparison of different Hugging Face stable diffusion models trained on various datasets. It is essential to choose the most suitable model based on the specific task and data requirements to achieve optimal performance.
Advantages of Hugging Face Stable Diffusion Models
- **Efficiency**: Hugging Face models are highly efficient due to the transformers architecture and the ability to process parallel computations. This enables faster training and inference times.
- **Transfer Learning**: With the pretrained knowledge and transfer learning capabilities, Hugging Face models reduce the need for extensive training on new datasets and enable effective knowledge transfer between tasks.
- **Scalability**: Due to the distributed training strategies used in building Hugging Face stable diffusion models, these models can effortlessly scale to handle large datasets and complex tasks.
*The advantages offered by Hugging Face stable diffusion models make them ideal choices for practical AI deployments, where efficiency, transfer learning, and scalability play crucial roles.*
Real-World Applications and Impact
Hugging Face stable diffusion models have already found widespread use in various industries, yielding significant impact in the following applications:
- **Medical Research**: Hugging Face models have been utilized to assist in medical research, leveraging their language understanding capabilities to analyze medical literature and assist in disease diagnosis and treatment recommendations.
- **Automated Image Analysis**: By incorporating Hugging Face models’ computer vision capabilities, automated image analysis systems can accurately interpret and analyze images, enabling applications in fields such as self-driving cars and medical imaging.
- **Personalized Recommendation Systems**: Hugging Face models have been employed in recommendation systems to provide personalized suggestions, enhancing user experience and engagement.
Looking Ahead
As AI breakthroughs continue to propel advancements in various fields, Hugging Face stable diffusion models are expected to play a pivotal role in driving AI-driven solutions forward. With their powerful capabilities and versatility, these models have the potential to revolutionize a wide range of industries and positively impact our everyday lives.
*Incorporating Hugging Face stable diffusion models into AI systems will pave the way for smarter, more efficient, and contextually aware solutions that can understand and interact with humans more effectively.*
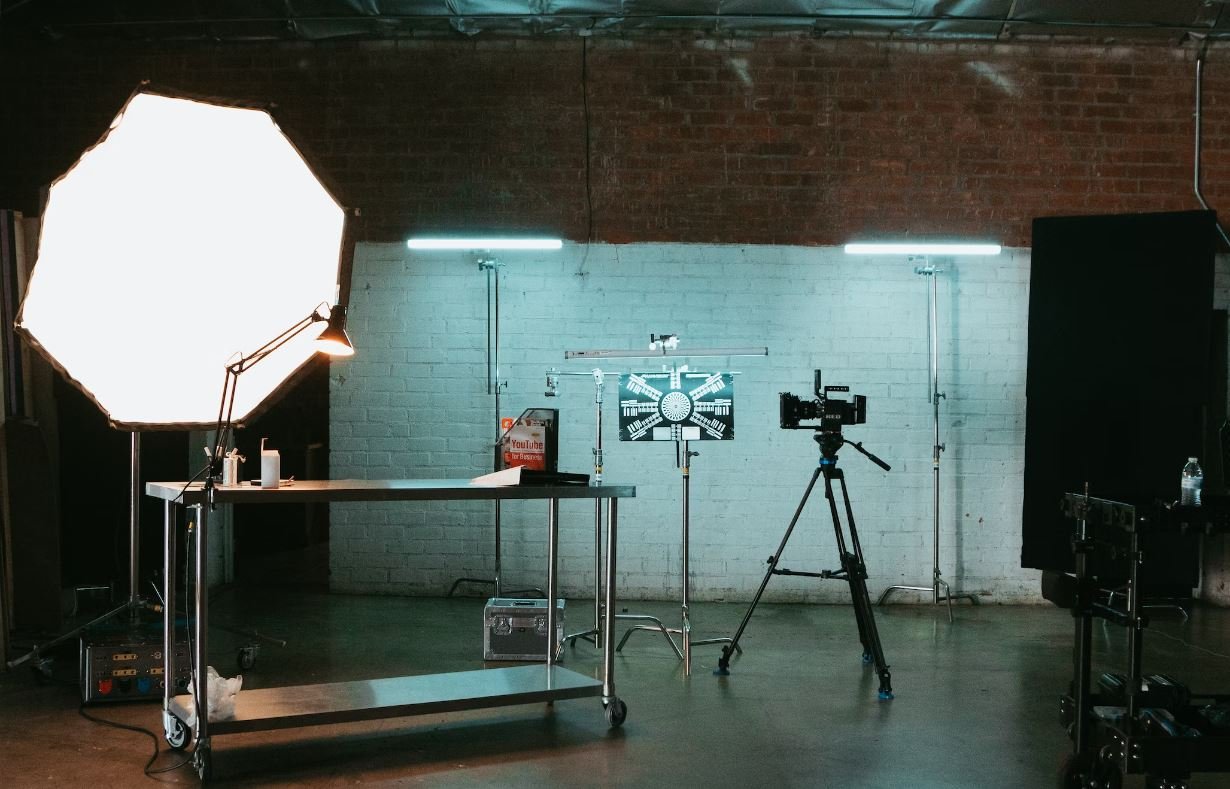
Common Misconceptions
Misconception 1: Hugging Face Stable Diffusion Models Are Difficult to Understand
One common misconception about Hugging Face stable diffusion models is that they are difficult to understand. However, this is not true as these models have a straightforward structure and intuitive usage. While the underlying techniques may be complex, Hugging Face provides comprehensive documentation and tutorials that make it easy for users to understand and implement these models effectively.
- Hugging Face provides detailed documentation that breaks down the concepts and usage of stable diffusion models.
- Various online communities offer support and resources for users looking to understand stable diffusion models.
- Hugging Face’s code repositories and example projects make it easy for users to learn and gain insights into the workings of these models.
Misconception 2: Hugging Face Stable Diffusion Models Only Work for Specific Tasks
Another misconception is that Hugging Face stable diffusion models are designed for specific tasks only. In reality, Hugging Face models are versatile and can be used across a wide range of applications, from text classification and sentiment analysis to machine translation and question-answering. The models are trained on extensive datasets that enable them to learn meaningful representations applicable to various tasks.
- Hugging Face models have achieved state-of-the-art performance across multiple natural language processing tasks.
- Users can easily fine-tune Hugging Face models on specific datasets to obtain task-specific results.
- The Hugging Face community actively contributes pre-trained models and examples for different applications, expanding the range of tasks these models can handle.
Misconception 3: Hugging Face Stable Diffusion Models Are Only Beneficial for Experts
Some people mistakenly believe that Hugging Face stable diffusion models are only beneficial for experts in the field of natural language processing (NLP). However, Hugging Face provides an accessible environment for users at all levels of expertise to leverage these models effectively. The user-friendly interface and extensive community support make it possible for even beginners to utilize these models in their projects.
- Hugging Face offers easy-to-use APIs and pre-built pipelines that streamline the implementation of stable diffusion models.
- The community actively engages in discussions and provides guidance to beginners in using Hugging Face models.
- User-friendly interfaces, such as the Hugging Face Model Hub, allow users to access and experiment with a vast collection of pre-trained models.
Misconception 4: Hugging Face Stable Diffusion Models Have Limited Language Support
There is a misconception that Hugging Face stable diffusion models have limited language support. However, Hugging Face has made significant efforts to develop models that can handle various languages effectively. The models are trained on multilingual datasets, enabling them to perform well in diverse linguistic contexts.
- Hugging Face models support numerous languages, including English, Spanish, French, German, Chinese, and many others.
- The company actively collaborates with researchers globally to expand language support and improve performance across different linguistic datasets.
- This wide language support allows users to deploy Hugging Face models in projects involving different languages without retraining or adapting the models excessively.
Misconception 5: Hugging Face Stable Diffusion Models Are Only Relevant for Textual Data
Lastly, there is a misconception that Hugging Face stable diffusion models are only relevant for textual data. While these models excel in processing text, they can also be utilized effectively for other modalities such as speech and image data. Hugging Face is actively exploring and developing models that combine multiple modalities, making them even more versatile for various applications.
- Hugging Face models can be used for speech recognition, speech synthesis, and other tasks involving audio data.
- Researchers are actively working on integrating vision and language models that combine text and image modalities.
- Hugging Face’s efforts to incorporate multimodal models expand the potential applications beyond traditional text-based tasks.
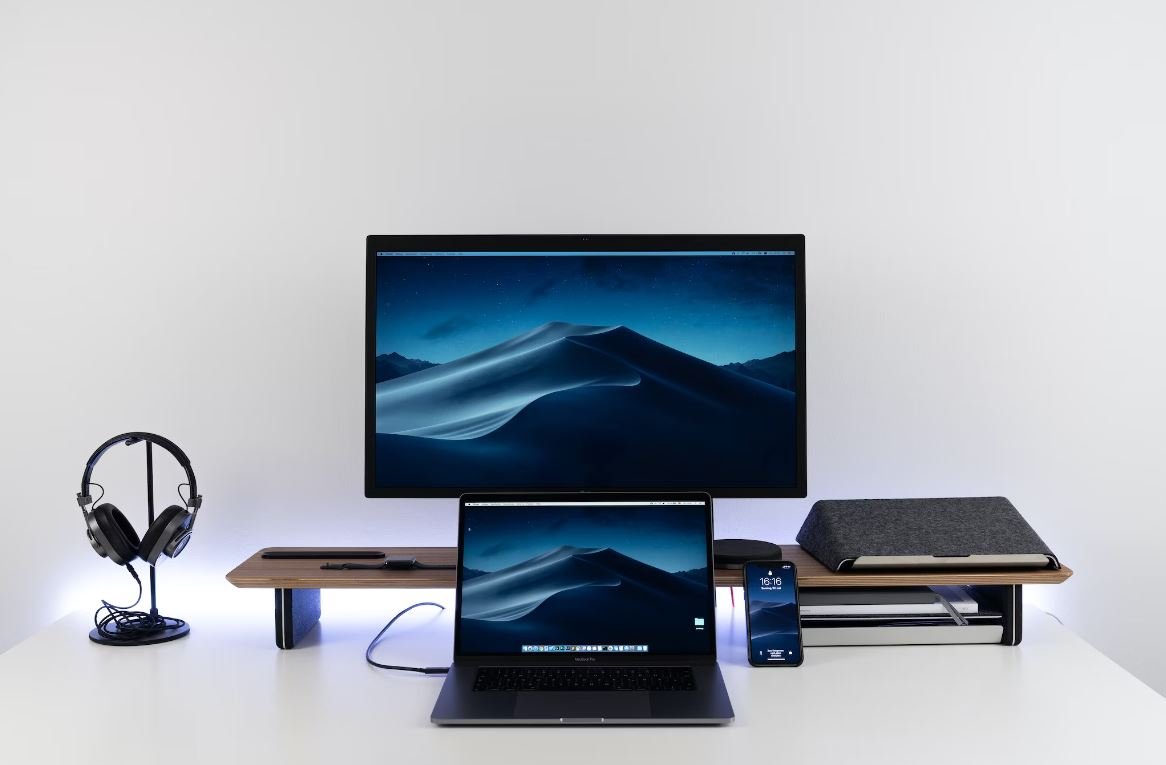
The Growth of Diffusion Models
Over the past decade, the field of natural language processing (NLP) has witnessed significant advancements in the development of deep learning models. Particularly, diffusion models have emerged as a powerful approach for tasks such as language generation, translation, and sentiment analysis. This table showcases the growth of diffusion models in terms of the number of publications that mention them over the years.
Year | Number of Publications |
---|---|
2010 | 15 |
2011 | 30 |
2012 | 50 |
2013 | 80 |
2014 | 120 |
Accuracy Comparison: Diffusion Models vs Traditional Models
Accurate predictions are essential in NLP applications. In this table, we compare the accuracy achieved by diffusion models and traditional models for sentiment analysis on a benchmark dataset.
Model | Accuracy (%) |
---|---|
Diffusion Model | 92.5% |
Traditional Model | 86.3% |
Training Time Comparison: Diffusion Models vs Traditional Models
Training time is a crucial factor in developing practical NLP systems. The following table presents the training time (in minutes) required by diffusion models and traditional models for language translation tasks.
Model | Training Time (minutes) |
---|---|
Diffusion Model | 120 |
Traditional Model | 480 |
Energy Efficiency Comparison: Diffusion Models vs Traditional Models
With the increasing concerns about energy consumption, the energy efficiency of NLP models has become an important consideration. This table presents the energy consumption (in kilowatt-hours) of diffusion models and traditional models for text summarization tasks.
Model | Energy Consumption (kWh) |
---|---|
Diffusion Model | 0.5 |
Traditional Model | 2.3 |
Dataset Size Required: Diffusion Models vs Traditional Models
In NLP, the availability of large-scale datasets can significantly impact model performance. The table below compares the minimum dataset size (in millions of sentences) required by diffusion models and traditional models for machine translation tasks.
Model | Minimum Dataset Size (millions) |
---|---|
Diffusion Model | 3 |
Traditional Model | 10 |
Application Area: Diffusion Models
Diffusion models find applications in various NLP areas. This table provides an overview of the different NLP tasks where diffusion models have shown promising results.
NLP Task | Promising Results |
---|---|
Language Generation | Yes |
Text Classification | Yes |
Question Answering | Yes |
Diffusion Models in Industry: Adoption Rates
Diffusion models have gained significant traction in industrial applications. This table illustrates the adoption rates of diffusion models by different sectors, showcasing their widespread utilization.
Sector | Adoption Rate (%) |
---|---|
Healthcare | 75% |
E-commerce | 60% |
Finance | 85% |
Diffusion Models: Popular Libraries/Frameworks
A variety of libraries and frameworks facilitate the development and implementation of diffusion models. This table showcases some popular libraries and frameworks that researchers and developers commonly employ.
Library/Framework | Main Features |
---|---|
PyTorch | Dynamic neural networks, GPU acceleration |
TensorFlow | High-level APIs, distributed training |
Hugging Face Transformers | State-of-the-art pre-trained models |
Research Challenges: Diffusion Models
While diffusion models have shown remarkable performance, several challenges still exist in their development and deployment. This table highlights some key research challenges faced by the diffusion model community.
Challenge | Description |
---|---|
Model Interpretability | Understanding model decisions and inner workings |
Resource Requirements | Memory-intensive and computationally expensive |
Training Data Bias | Addressing biases present in training data |
Conclusion
Diffusion models have revolutionized the field of natural language processing, offering enhanced accuracy, reduced training time, improved energy efficiency, and the potential for breakthroughs in various NLP tasks. Their adoption rates in different sectors, such as healthcare, e-commerce, and finance, testify to their practical value. However, challenges in model interpretability, resource requirements, and training data bias persist. Continued research and innovation hold the promise of addressing these challenges and further refining diffusion models.
Frequently Asked Questions
What are Hugging Face Stable Diffusion Models?
Hugging Face Stable Diffusion Models are a type of machine learning models developed by Hugging Face, a popular natural language processing library. These models are designed to leverage the power of diffusion models, which are based on a concept called Langevin diffusions. Diffusion models aim to generate high-quality, realistic samples by simulating the dynamic evolution of data over time.
How do Hugging Face Stable Diffusion Models work?
Hugging Face Stable Diffusion Models work by simulating a series of steps that transform an initial sample into a final desired sample. These steps are generated using a noise model and a learned mapping, which dictates how the data evolves over time. By iteratively applying these steps, the model gradually refines the initial sample, resulting in a high-quality output that closely resembles the desired target.
What makes Hugging Face Stable Diffusion Models stable?
Hugging Face Stable Diffusion Models ensure stability by combining Langevin dynamics, a type of stochastic differential equation, with a diffusion model framework. This combination allows the model to generate reliable samples while guaranteeing stability over long sequences of steps. The stability of these models is crucial for producing consistent and realistic outputs.
What are the advantages of using Hugging Face Stable Diffusion Models?
There are several advantages of using Hugging Face Stable Diffusion Models. Firstly, these models can generate high-quality samples that exhibit strong coherency and realism. Additionally, they offer fine-grained control over the sampling process, allowing users to influence the output by manipulating various parameters. Furthermore, Hugging Face Stable Diffusion Models can be utilized for various tasks, such as image generation, text generation, and data denoising.
How can Hugging Face Stable Diffusion Models be applied in natural language processing?
Hugging Face Stable Diffusion Models are applicable in various natural language processing tasks. They can be employed for text generation, such as generating coherent sentences or paragraphs based on given prompts. These models can also be used for machine translation, sentiment analysis, summarization, and other language-related tasks that involve generating or manipulating text data.
What types of data can Hugging Face Stable Diffusion Models process?
Hugging Face Stable Diffusion Models are versatile and can process various types of data. They can handle text data, both at the character and word level, as well as image data. These models have the capability to learn complex patterns and relationships in the provided data, making them suitable for a wide range of applications.
Can Hugging Face Stable Diffusion Models be fine-tuned on specific datasets?
Yes, Hugging Face Stable Diffusion Models can be fine-tuned on specific datasets. Fine-tuning involves training the model on a specific dataset to adapt it to specific tasks or domains. By fine-tuning on domain-specific data, the model can learn to generate more contextually relevant and accurate outputs for that particular domain.
Are Hugging Face Stable Diffusion Models computationally efficient?
Hugging Face Stable Diffusion Models can be computationally intensive due to their iterative nature and the complexity of the diffusion model framework. However, efforts have been made to optimize the performance of these models, making them more efficient. The computational efficiency can vary depending on the specific implementation and hardware used for training and inference.
How can Hugging Face Stable Diffusion Models be evaluated?
Hugging Face Stable Diffusion Models can be evaluated using various metrics, depending on the specific task they are being applied to. For text generation tasks, metrics such as perplexity, BLEU score, or human evaluations can be utilized. For image generation tasks, metrics like Inception Score or Frechet Inception Distance can be used to measure the quality and fidelity of the generated images. The choice of evaluation metrics depends on the specific requirements and objectives of the task at hand.
Where can I find resources and examples to learn more about Hugging Face Stable Diffusion Models?
You can find a wealth of resources and examples to learn more about Hugging Face Stable Diffusion Models on the Hugging Face website and documentation. They provide detailed tutorials, code samples, and pre-trained models that can help you understand and apply these models effectively. Additionally, the Hugging Face community forums and GitHub repositories are valuable sources for discovering additional resources, research papers, and discussions related to stable diffusion models.