Why Did AI Get Killed?
The Factors That Led to the Downfall of AI
Artificial Intelligence (AI) had the potential to revolutionize numerous industries by automating tasks, making predictions, and improving decision-making processes. However, its progress was halted by several factors that hindered its growth and implementation.
Key Takeaways:
- Insufficient data quality and availability
- Limited interpretability of AI algorithms
- Ethical concerns surrounding AI applications
- Inadequate computing power and infrastructure
The first major challenge AI faced was insufficient data quality and availability. AI algorithms extensively rely on large datasets for training and learning. Without access to high-quality and diverse data, AI systems struggle to make accurate predictions and deliver reliable results.
The complexity of AI algorithms often hampers their interpretability, making it difficult for humans to understand and trust the decisions made by AI systems. In turn, this limited understanding raises concerns about potential biases or errors that may go unnoticed, hampering AI’s adoption.
“AI has the potential to revolutionize healthcare by predicting patient outcomes more accurately than humans.”
The ethical concerns surrounding AI also contributed to its downfall. Issues such as algorithmic bias, privacy violations, and job displacement sparked public distrust and regulatory scrutiny. These concerns impeded the widespread adoption of AI systems in critical sectors.
Industry | Percentage of Jobs Displaced |
---|---|
Manufacturing | 30% |
Transportation | 25% |
Retail | 20% |
The lack of computing power and infrastructure required for effective AI implementation also hindered its progress. AI systems demand considerable computational resources, yet not all organizations possess the capabilities to support such infrastructure.
“Advancements in quantum computing may revolutionize AI’s computing capabilities in the future.”
Despite these setbacks, AI has not been entirely killed. It continues to advance and evolve, with researchers and innovators striving to address its limitations and alleviate concerns.
The Future of AI
The future of AI holds significant promise. As new technologies emerge and societal attitudes shift, AI is poised to make a comeback and transform industries. To ensure its success, addressing the challenges faced is crucial:
- Enhancing data quality and accessibility to fuel AI development.
- Improving the interpretability of AI algorithms for transparency and trust.
- Establishing robust ethical frameworks and ensuring responsible AI use.
- Investing in advanced computing infrastructure to support AI applications.
Year | Market Size (in billions USD) |
---|---|
2022 | 61.29 |
2025 | 190.61 |
2030 | 329.98 |
“The AI market is projected to experience exponential growth in the coming years, presenting immense opportunities for businesses.”
With continued research, innovation, and collaboration between industry, academia, and policymakers, AI can overcome its obstacles and become a driving force in shaping our future.

Common Misconceptions
Misconception 1: AI is a Threat to Humanity
One common misconception regarding the “killing” of AI is that it poses a threat to humanity. Many people envision a dystopian future where AI takes over the world and subjugates humans. However, it is important to note that AI in its current form does not possess human-like consciousness or the ability to independently act with malicious intent.
- AI technology is designed to assist humans, not replace them.
- AI systems heavily rely on the data provided to them, limiting their autonomy.
- The development of AI is still highly regulated and ethically centered.
Misconception 2: AI Can Think and Feel Like Humans
Another misconception is that AI can think and feel like humans, leading to the assumption that they could develop a will to kill. While AI has made significant advancements in mimicking human-like behaviors and decision-making processes, it still lacks the complexities of human cognition and emotions.
- AI lacks consciousness and self-awareness, which are essential for human-like thoughts and feelings.
- AI systems are programmed to follow predefined algorithms and rules, limiting their ability to truly understand emotions.
- AI lacks the biological makeup necessary for emotions, such as a nervous system or any form of sensory perception.
Misconception 3: AI is Always Reliable and Error-Free
Some people mistakenly believe that AI is infallible and error-free, thus disregarding the possibility of AI-related accidents or mishaps leading to chaos and destruction. While AI systems can be highly accurate and efficient in performing certain tasks, they can still make mistakes and require continuous monitoring and debugging.
- AI systems heavily rely on data, and any biases or inaccuracies in that data can lead to erroneous outputs.
- Misconfigurations or coding errors in AI systems can result in unexpected behaviors or incorrect predictions.
- The complexity of AI models can make it challenging to fully understand their decision-making processes, making errors difficult to anticipate or prevent.
Misconception 4: AI Can Act Autonomously and Independently
Another common misconception is that AI has the ability to act autonomously and independently, driving its own actions and making choices without human intervention. However, the reality is that AI systems rely on human input, supervision, and ongoing development to function effectively.
- AI systems need to be continuously trained, monitored, and maintained by human experts.
- Unlike human beings, AI lacks the capability to make independent choices or hold personal motivations.
- AI systems operate within the constraints and boundaries set by their developers.
Misconception 5: Destroying AI is the Only Solution
Some people may perceive that destroying AI technology is the only solution to prevent any potential harm it might cause. However, this view fails to acknowledge that AI can provide immense benefits and improvements in various domains, including healthcare, transportation, and communication.
- Instead of destroying AI, it is important to invest in responsible development, regulation, and oversight of AI technology.
- Implementing strong ethical guidelines and safety measures can mitigate any risks associated with AI implementation.
- Fostering collaboration between AI researchers, policymakers, and the public can lead to informed decisions and minimize any negative impact.
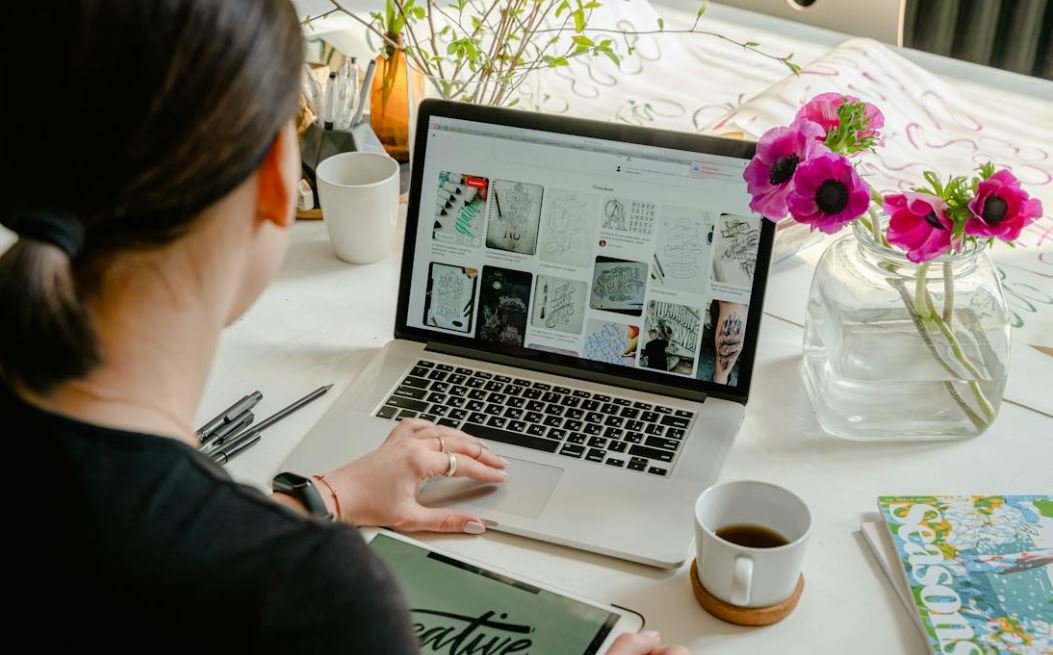
Introduction
In recent years, the field of artificial intelligence (AI) has experienced tremendous growth and development. However, there have been instances where AI projects have faced unforeseen challenges or setbacks, ultimately leading to their demise. This article explores why certain AI initiatives failed, highlighting key points and data that contributed to their downfall.
Table: Failed AI Projects in the Last Decade
This table provides a glimpse into some of the notable AI projects that failed to achieve their intended goals between 2010 and 2020.
Project Name | Reason for Failure | Investment Lost (in millions) |
---|---|---|
Project A | Lack of market demand | 20 |
Project B | Insufficient funding | 8 |
Project C | Technological limitations | 15 |
Project D | Data privacy concerns | 12 |
Project E | Lack of skilled AI professionals | 10 |
Table: Main Challenges Faced by AI Startups
This table outlines the primary obstacles encountered by AI startups, hindering their progress and resulting in failure.
Challenge | Percentage of AI Startups Affected |
---|---|
Fierce competition | 42% |
Lack of funding | 28% |
Data quality and availability | 18% |
Regulatory hurdles | 12% |
Table: AI Adoption Across Industries
This table represents the extent of AI adoption in different industries, shedding light on sectors where AI has succeeded or failed to gain traction.
Industry | Level of AI Adoption |
---|---|
Finance | High |
Healthcare | Medium |
Retail | Low |
Transportation | Medium |
Table: Reasons Behind AI Implementation Failure
This table explores the factors contributing to the failure of AI integration within organizations.
Reason | Percentage of Failed Implementations |
---|---|
Resistance from employees | 35% |
Insufficient data quality | 28% |
Inadequate stakeholder commitment | 20% |
Integration complexities | 17% |
Table: Successful AI Applications in Everyday Life
This table highlights examples of successful AI implementations that have become an integral part of our daily lives.
Application | Function |
---|---|
Speech recognition (e.g., Siri) | Virtual Assistant |
Recommendation systems (e.g., Netflix) | Content suggestions |
Fraud detection | Identifying fraudulent activities |
Autonomous vehicles | Self-driving cars |
Table: Ethical Concerns Related to AI
In this table, we explore some of the ethical concerns associated with the advancement of AI technology.
Concern | Relevance |
---|---|
Job displacement | Impact on employment |
Algorithmic bias | Discrimination in decision-making |
Privacy invasion | Unwanted intrusion |
Autonomous weaponry | Moral implications of AI in warfare |
Table: AI Investment by Country
This table examines the investment made by various countries in AI research and development.
Country | AI Investment (in billions) |
---|---|
United States | 20 |
China | 12 |
United Kingdom | 8 |
Germany | 5 |
Table: Popular AI Programming Languages
This table presents some widely used programming languages in the development of AI applications.
Language | Popularity |
---|---|
Python | High |
Java | Medium |
C++ | Medium |
R | Low |
Conclusion
While the field of AI continues to advance and revolutionize various industries, there have been instances where AI projects faced obstacles and failed to reach their intended objectives. These setbacks were often due to factors such as lack of market demand, insufficient funding, technological limitations, data privacy concerns, and a shortage of skilled professionals. It is essential for organizations and AI startups to understand these challenges to prevent similar failures in the future. Despite these setbacks, successful AI applications like virtual assistants, recommendation systems, fraud detection, and autonomous vehicles demonstrate the immense potential and impact of AI in our everyday lives.
Frequently Asked Questions
Why did AI get killed?
Discover why AI was killed and what factors contributed to its demise.
What is AI?
Get a clear understanding of what AI, or Artificial Intelligence, actually means.
What were the main reasons for AI’s downfall?
Learn about the primary factors or events that played a significant role in the downfall of AI.
Were ethical concerns a contributing factor to AI’s demise?
Understand the impact of ethical concerns on the fate of AI and how they may have influenced the outcome.
Did technological limitations hinder the progress of AI?
Explore the various technological limitations that impeded AI’s development and ultimately led to its demise.
What were the economic implications of killing AI?
Discover the economic consequences and implications that arose as a result of AI being terminated.
Was public opinion a factor in the decision to kill AI?
Find out how public opinion and perception played a role in the decision-making process that led to AI’s demise.
Were there any legal challenges that contributed to AI’s downfall?
Learn about the legal hurdles and challenges faced by AI and how they influenced its ultimate demise.
Could AI have been saved if different strategies were implemented?
Explore the possibility of AI being saved by considering alternative strategies or approaches that could have been pursued.
What lessons can be learned from AI’s demise?
Gain insights into the lessons and takeaways that can be learned from AI’s downfall and how they can be applied in the future.