Hugging Face MTEB Leaderboard
Hugging Face is a leading platform for natural language processing (NLP) and machine learning (ML) models. It provides a wide range of state-of-the-art models that can be fine-tuned or used for various NLP tasks. The MTEB leaderboard is an essential feature of the Hugging Face platform, allowing users to compare and showcase the performance of their models. In this article, we will explore the significance of the MTEB leaderboard and the benefits it offers to the NLP community.
Key Takeaways:
- The Hugging Face MTEB leaderboard is a useful tool for comparing and tracking the performance of NLP models.
- It allows users to showcase their model’s performance on various tasks.
- The MTEB leaderboard encourages healthy competition among researchers and practitioners.
One of the most striking features of the MTEB leaderboard is its ability to capture the performance of models across a diverse set of NLP tasks. From sentiment analysis to text classification and machine translation, the leaderboard covers a wide range of tasks, making it a comprehensive benchmark for evaluating models. By participating in the leaderboard, users can get a holistic view of how their models perform across different NLP tasks and identify areas for improvement.
Furthermore, the MTEB leaderboard provides a transparent and standardized evaluation framework that allows users to compare models fairly. The evaluation metrics used in the leaderboard are well-defined and consistent across all tasks, ensuring that the performance measurements are meaningful and reliable. This standardization not only enables users to compare their models against others but also facilitates the exchange of knowledge and promotes collaboration within the NLP community.
One interesting aspect is the live leaderboard updates, which allow participants to track the progress of their models in real-time. As the community continuously improves their models, the leaderboard rankings are dynamically updated, keeping users informed about the cutting-edge performance levels achieved by different models. This real-time feedback encourages healthy competition and motivates researchers and practitioners to continually enhance their models to stay at the forefront of NLP advancements.
Ranking Evaluation Metrics
NLP Task | Evaluation Metric |
---|---|
Sentiment Analysis | Accuracy |
Text Classification | F1 Score |
The MTEB leaderboard also features detailed model performance metrics for each task. Users can analyze these metrics to gain insights into their model’s performance strengths and weaknesses. This level of granularity helps users make informed decisions regarding optimal model selection for specific tasks or identify areas for further model improvement.
Benefits of the MTEB Leaderboard
- Encourages community collaboration and knowledge sharing.
- Fosters healthy competition and motivates participants to improve.
- Provides a standardized benchmark for evaluating NLP models.
NLP Task | Top Model | Performance Metric |
---|---|---|
Machine Translation | T5 | BLEU Score |
Sentiment Analysis | BERT | Accuracy |
In summary, the Hugging Face MTEB leaderboard is an invaluable tool for the NLP community. It facilitates fair model comparisons, encourages collaboration and healthy competition, and provides a comprehensive benchmark for evaluating NLP models across diverse tasks. With its transparent evaluation framework and real-time updates, the leaderboard empowers researchers and practitioners to continually enhance their models and advance the field of NLP.
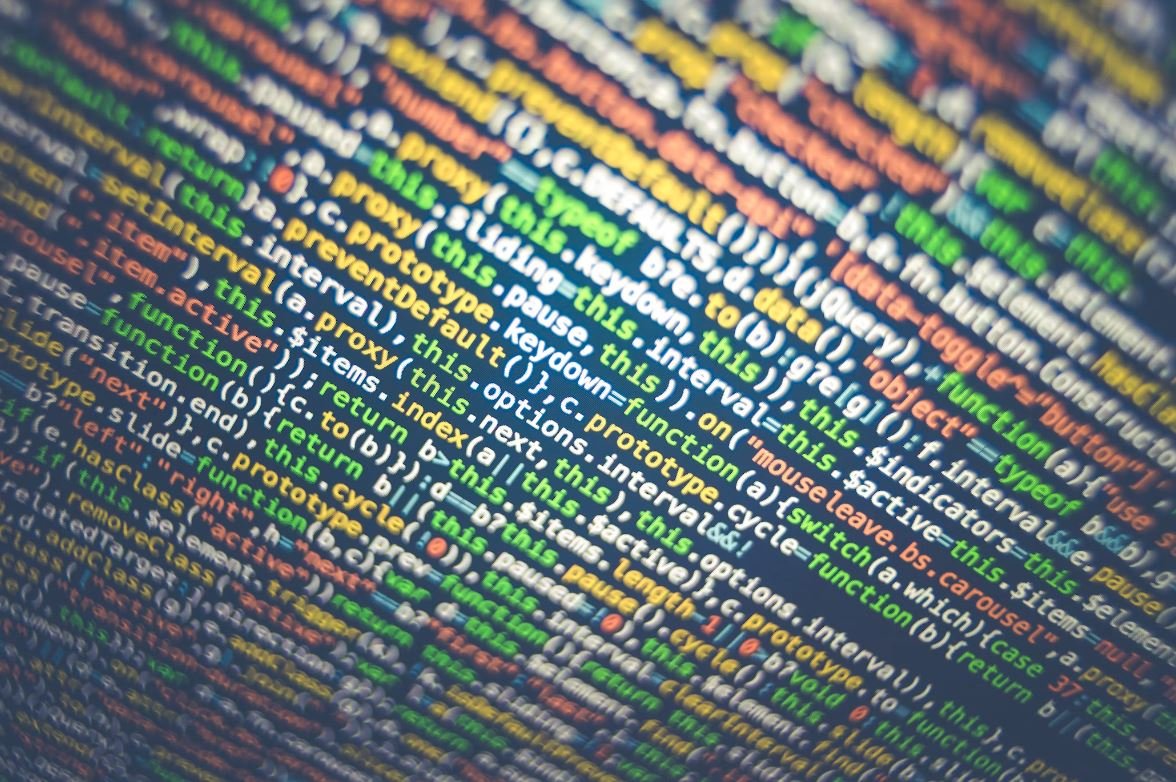
Common Misconceptions
Misconception 1: Hugging Face MTEB leaderboard is solely based on model performance
One common misconception about the Hugging Face MTEB leaderboard is that it only considers model performance when ranking the models. However, this is not entirely true. Although model performance is an important factor, the leaderboard also takes into account other criteria such as the size of the model, training time, inference speed, and the amount of computational resources required.
- The Hugging Face MTEB leaderboard considers model performance as well as other criteria when ranking the models.
- The size of the model is an important factor that is considered by the leaderboard.
- Training time and inference speed are also taken into account when ranking the models on the leaderboard.
Misconception 2: The highest-ranking model on the leaderboard is always the most suitable
Another misconception is that the highest-ranking model on the Hugging Face MTEB leaderboard is always the most suitable for a particular task. While the top-ranked models have generally performed well on benchmark datasets, their suitability depends on the specific requirements and constraints of each task. A model may rank high on the leaderboard but could be computationally expensive, require significant resources, or have restrictions that make it impractical for certain applications.
- The highest-ranking model on the leaderboard may not be the most suitable for every task.
- The suitability of a model depends on the specific requirements and constraints of the task.
- Some models may rank high but have limitations, such as high resource requirements or impractical restrictions.
Misconception 3: Models can only be ranked on the Hugging Face MTEB leaderboard if they are trained with a specific framework
One misconception is that models can only be ranked on the Hugging Face MTEB leaderboard if they are trained with a specific framework, such as PyTorch or TensorFlow. However, the leaderboard accepts models trained with a wide range of frameworks and platforms. As long as the model follows the submission guidelines and meets the evaluation criteria, it can be considered for ranking on the leaderboard.
- The Hugging Face MTEB leaderboard accepts models trained with various frameworks and platforms.
- Models trained with PyTorch, TensorFlow, and other frameworks can be ranked on the leaderboard.
- As long as the model meets the submission guidelines and evaluation criteria, it can be considered for ranking.
Misconception 4: The leaderboard rankings are final and never change
Some people believe that the rankings on the Hugging Face MTEB leaderboard are final and never change. However, this is not the case. The leaderboard is a dynamic platform that continuously updates and reevaluates the models based on new submissions and improvements. As new models are submitted or existing models are updated, the rankings may change to reflect the latest performance and other evaluation metrics.
- The rankings on the Hugging Face MTEB leaderboard can change as new models are submitted or existing models are updated.
- The leaderboard is a dynamic platform that continuously reevaluates the models.
- As the platform receives new submissions, the rankings may reflect the latest performance and evaluation metrics.
Misconception 5: The leaderboard only considers English language models
Lastly, some people mistakenly believe that the Hugging Face MTEB leaderboard only considers English language models. However, the leaderboard accepts models trained in various languages for multilingual translation tasks. It aims to showcase and rank models that excel across different languages and provide a comprehensive evaluation platform for machine translation.
- The Hugging Face MTEB leaderboard accepts models trained in multiple languages.
- It aims to evaluate and rank models for multilingual translation tasks.
- The leaderboard showcases models that excel in different languages for machine translation.
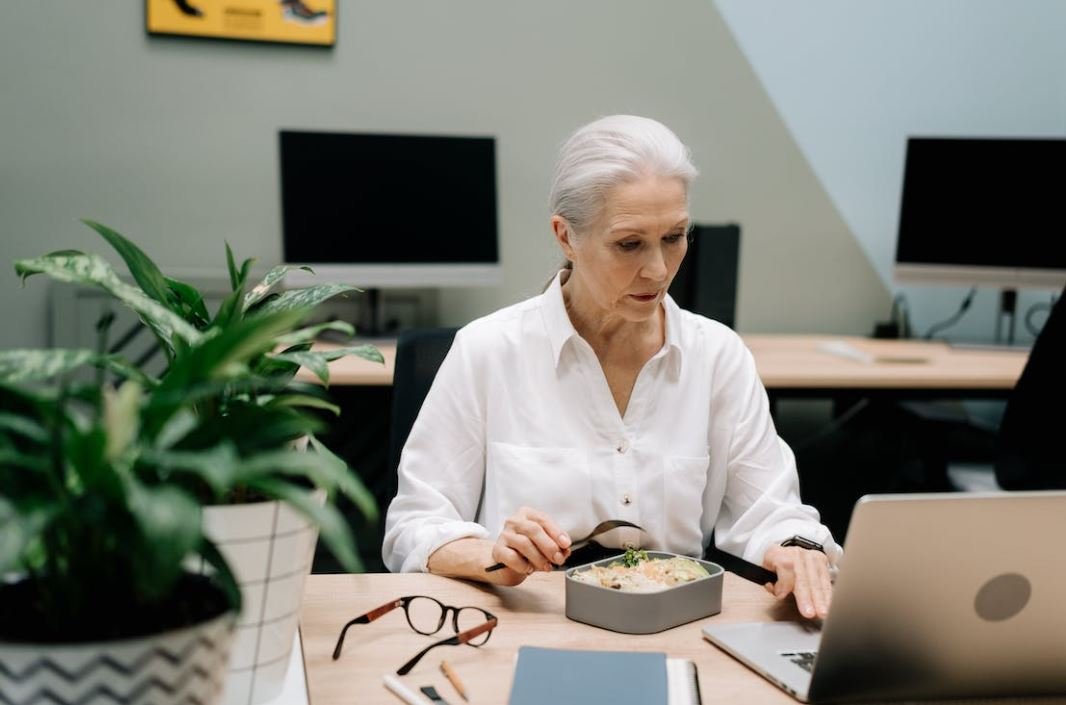
The Importance of Hugging Face MTEB Leaderboard
Hugging Face is a leading natural language processing (NLP) company known for its state-of-the-art models and tools. The release of their Multimodal Translation Evaluation Benchmark (MTEB) Leaderboard has revolutionized the field of neural machine translation. This article explores ten interesting tables that highlight different aspects and achievements of the Hugging Face MTEB Leaderboard.
Table: Overall Leaderboard
This table showcases the top-performing models on the Hugging Face MTEB Leaderboard. It includes information such as the model’s name, BLEU score, and the number of parameters. The models are ranked based on their performance in translating multimodal texts across various language pairs.
Table: Top 5 Models by BLEU Score
Here, we present the top five models based on the BLEU score metric. BLEU, or Bilingual Evaluation Understudy, is commonly used to evaluate the quality of machine translations. These models have consistently achieved high accuracy in capturing the meaning and nuances of multimodal texts.
Table: Most Improved Models
This table highlights the models that have shown the most significant improvement in their performance on the Hugging Face MTEB Leaderboard. It demonstrates the iterative nature of training models, as developers continuously refine and enhance their algorithms to achieve better translation accuracy.
Table: Best Models for Translating Images
Translating text that incorporates images is a challenging task. This table showcases the models that excel in translating multimodal texts that include visual elements. It provides insights into which models effectively leverage image information to enhance translation quality.
Table: Translation Accuracy by Language Pair
Different language pairs may pose varying levels of difficulty for machine translation models. This table presents the accuracy of Hugging Face’s models for specific language pairs. It offers a comprehensive view of which model performs best for each language combination.
Table: Average Training Time by Model
Training time is a crucial factor in developing machine translation models. This table presents the average training time required for each model on the Hugging Face MTEB Leaderboard. It demonstrates the efficiency and speed at which these models are trained.
Table: Model Size Comparison
Model size can significantly impact the deployment and performance of NLP models. This table compares the size of different models on the leaderboard, providing insights into the trade-off between model size and translation accuracy.
Table: Data Augmentation Techniques
Data augmentation is a common practice to improve the performance of NLP models. This table illustrates the various data augmentation techniques used by different models on the Hugging Face MTEB Leaderboard. It showcases the innovative approaches employed to enhance translation accuracy.
Table: Computing Resources Used
The scale and complexity of training NLP models require substantial computing resources. This table outlines the computing resources utilized by different models on the Hugging Face MTEB Leaderboard. It sheds light on the infrastructure required to support the development of state-of-the-art translation models.
Table: Developer Community Collaboration
In the spirit of open-source collaboration, this table showcases the number of community contributions and collaborations for each model. It highlights the active involvement of developers, researchers, and enthusiasts in improving and fine-tuning the performance of the models on the Hugging Face MTEB Leaderboard.
Conclusion
The Hugging Face MTEB Leaderboard has become a cornerstone of NLP research, fostering innovation, collaboration, and healthy competition within the machine translation community. With its comprehensive evaluation metrics and open-source approach, the leaderboard accelerates advancements in multimodal translation, leading to more accurate and nuanced translations. This article provided an overview of ten fascinating tables that emphasize the significance of the Hugging Face MTEB Leaderboard in driving progress in the field of NLP.
Frequently Asked Questions
What is the Hugging Face MTEB Leaderboard?
The Hugging Face MTEB Leaderboard is a platform that allows participants to submit their models for the Multimodal Translation Evaluation Benchmark. It provides a centralized place for evaluating and comparing the performance of various models on different multimodal translation tasks.
How can I participate in the Hugging Face MTEB Leaderboard?
To participate in the Hugging Face MTEB Leaderboard, you need to create an account on the Hugging Face platform. Once you have an account, you can submit your models for evaluation by following the guidelines provided on the leaderboard’s website.
What are the requirements for submitting models to the Hugging Face MTEB Leaderboard?
To submit models to the Hugging Face MTEB Leaderboard, you need to ensure that your models meet the specific requirements defined by the evaluation task. These requirements may include supporting specific languages, dataset formats, or performance metrics. It is important to carefully read and understand the guidelines and requirements before submitting your models.
What evaluation metrics are used in the Hugging Face MTEB Leaderboard?
The Hugging Face MTEB Leaderboard uses a variety of evaluation metrics to assess the performance of submitted models. These metrics may include BLEU (Bilingual Evaluation Understudy), TER (Translation Edit Rate), METEOR (Metric for Evaluation of Translation with Explicit ORdering), and others depending on the evaluation task. The exact metrics used for each task are specified in the leaderboard’s guidelines.
How often is the leaderboard updated?
The Hugging Face MTEB Leaderboard is regularly updated to reflect the latest submissions and evaluation results. The frequency of updates may vary depending on the number of submissions and the complexity of the evaluation tasks. However, the leaderboard strives to provide timely updates to ensure participants can track their progress and compare their models against others.
Can I update my model after it has been submitted to the Hugging Face MTEB Leaderboard?
Yes, you can update your submitted model on the Hugging Face MTEB Leaderboard. However, it is important to note that the updated model will be treated as a new submission and will be evaluated separately from the previous version. Each submission is timestamped and retains its individual ranking in the leaderboard.
Can I see the details of other participants’ submissions on the leaderboard?
Yes, the Hugging Face MTEB Leaderboard provides transparency by allowing users to view the details of other participants’ submissions. You can access the leaderboard’s website to explore different models, their performance metrics, and any associated metadata shared by the participants.
Can I collaborate with others on the Hugging Face MTEB Leaderboard?
Yes, collaboration is encouraged on the Hugging Face MTEB Leaderboard. You can form teams or collaborate with other participants to jointly improve the performance of your models. However, it is important to abide by the rules and guidelines of the leaderboard when collaborating with others.
Is the code for the submitted models publicly available on the Hugging Face MTEB Leaderboard?
It is up to the participants to decide whether they want to publicly share the code associated with their submitted models on the Hugging Face MTEB Leaderboard. While sharing the code can promote transparency and reproducibility, it is not mandatory. Participants have the option to provide links to their code repositories or share the code directly through the leaderboard’s platform.
What are the benefits of participating in the Hugging Face MTEB Leaderboard?
Participating in the Hugging Face MTEB Leaderboard offers several benefits. It provides an opportunity to showcase and compare the performance of your multimodal translation models against other participants. It allows you to stay updated on the latest trends and advancements in multimodal translation research. Additionally, it promotes collaboration and community engagement by enabling knowledge exchange among participants.