AI vs. Machine Learning: Understanding the Differences
Artificial Intelligence (AI) and Machine Learning (ML) are two buzzwords that are often used interchangeably, leading to confusion among many. While the terms are related, they refer to different concepts that have distinct implications and applications. In this article, we will delve into the differences between AI and ML, providing you with a clear understanding of these fascinating technologies.
Key Takeaways:
- AI and ML are related but distinct concepts.
- AI refers to the broader concept of machines exhibiting human-like intelligence, while ML is a subset of AI focused on enabling machines to learn from data.
- AI can be both narrow (performing specific tasks) or general (exhibiting human-like intelligence across various domains).
- ML involves algorithms that allow machines to learn patterns and make decisions without being explicitly programmed.
**Artificial Intelligence**, as a concept, aims to create machines that can perform tasks that typically require human intelligence. It encompasses a broad spectrum of capabilities, ranging from basic rule-based systems to sophisticated problem-solving systems. **Machine Learning**, on the other hand, is a subset of AI that focuses on enabling machines to learn from data without being explicitly programmed.
*Machine Learning* algorithms **automatically learn and improve** from experience without being programmed explicitly.
One way to understand the relationship between AI and ML is to think of AI as the broader umbrella, under which ML resides. While AI includes other techniques like expert systems and natural language processing, ML is specifically concerned with statistical algorithms that enable machines to learn from and make predictions or take actions based on data.
**To illustrate the differences between AI and ML, let’s examine three key areas:**
Table 1: AI vs. ML vs. Human Intelligence
AI | ML | Human Intelligence | |
---|---|---|---|
Capabilities | Varies from narrow to general intelligence | Can learn from data and make predictions or take actions | Complex problem-solving, sense-making, creativity, emotional intelligence, etc. |
Programming | Explicitly programmed and rules-based | Algorithms learn and improve without explicit programming | Demonstrated through learning and experiences |
Data Dependency | May or may not require data | Relies on data for learning and decision-making | Combined with knowledge and experiences |
**Narrow AI** refers to AI systems that are designed to perform specific tasks, such as image recognition or language translation. These systems operate within a constrained domain and are generally not capable of exhibiting intelligence outside of their assigned tasks. **General AI**, on the other hand, aims to mimic human intelligence across various domains, exhibiting both breadth and depth in problem-solving capabilities.
*Machine Learning*, as a subset of AI, **trains models by exposing them to vast amounts of data**, allowing them to learn patterns and make predictions or decisions based on that data. This capability has applications in numerous fields, from self-driving cars and recommendation systems to fraud detection and medical diagnosis.
**Table 2: Traditional Programming vs. Machine Learning Approach**
*Traditional Programming* | *Machine Learning* | |
---|---|---|
Programming Approach | Programmer writes explicit rules and instructions | Machine learns from data to infer rules and patterns |
Required Data | Not data-dependent | Large amounts of labeled data for training |
Flexibility | Less flexible in adapting to new or changing scenarios | Capable of adapting and improving with new data |
***Deep Learning**, a subfield of ML inspired by the human brain’s neural networks, is gaining significant attention. It has driven advancements in fields such as computer vision and natural language processing by enabling machines to learn hierarchical representations of data.*
In the *competitive landscape of AI and ML*, where businesses and industries are constantly seeking a competitive edge, it is crucial to understand the differences between these technologies to make informed decisions about their applications. While both AI and ML have transformative potential, it is important to recognize that AI encompasses broader capabilities beyond ML.
**Table 3: AI vs. ML Use Cases**
AI Use Cases | ML Use Cases | |
---|---|---|
Image Recognition | Facial recognition, object detection | Image labeling, image generation |
Natural Language Processing | Chatbots, language translation | Sentiment analysis, text generation |
Autonomous Vehicles | Self-driving cars | Decision-making, control systems |
**In summary**, Artificial Intelligence and Machine Learning are distinct yet related concepts. AI encompasses a broader range of capabilities, including ML, while ML focuses specifically on enabling machines to learn from data. ML enables machines to make predictions or decisions by learning patterns from the provided data. With ongoing advancements in these fields, AI and ML continue to revolutionize various industries, offering endless possibilities for innovation and problem-solving.
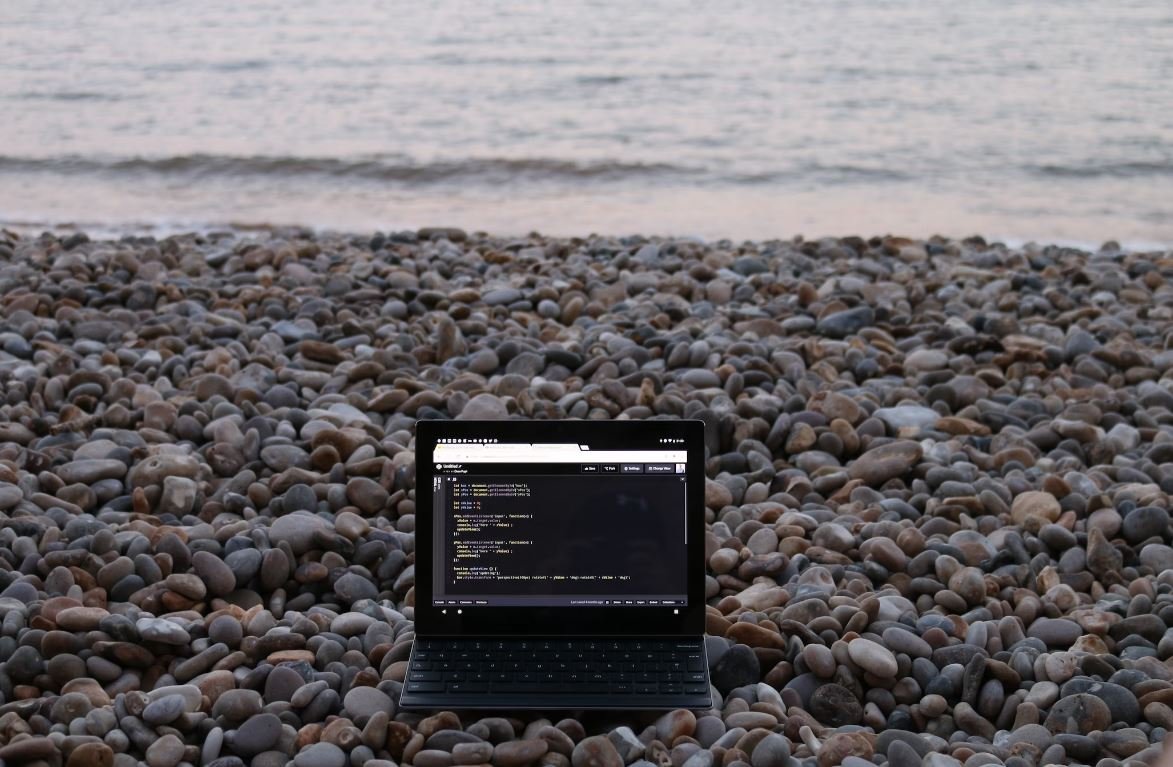
Common Misconceptions
1. Humans will be replaced by AI
One common misconception is that AI will completely replace humans in various fields. While AI technology has advanced significantly, it is important to understand that AI is designed to assist rather than replace humans.
- AI systems rely on human input to function effectively.
- Human creativity, judgment, and empathy cannot be easily replicated by AI.
- AI is more likely to complement human skills, leading to increased efficiency and productivity.
2. AI will always make objective and unbiased decisions
Another misconception is that AI is always objective and unbiased. However, AI models are trained on existing data, which can contain biases. As a result, AI systems can inadvertently perpetuate or amplify existing biases.
- AI algorithms may learn biases present in training data.
- AI models need to be carefully monitored and updated to mitigate biases and ensure fairness.
- The responsibility for making ethical decisions still lies with human operators, even when assisted by AI.
3. AI will take over all jobs
There is a fear that AI will lead to widespread unemployment. However, while AI may automate certain tasks, it is unlikely to completely replace all jobs.
- AI is more likely to augment existing jobs rather than eliminate them entirely.
- AI can create new opportunities in emerging fields related to AI development and maintenance.
- Certain jobs, such as those requiring creativity, emotional intelligence, and complex decision-making, are less likely to be replaced by AI.
4. AI is only for tech-savvy individuals
Many people believe that AI is only accessible to those with strong technical skills. However, AI technology is becoming more user-friendly and accessible to a wider range of individuals and industries.
- AI platforms and tools are being developed with user-friendliness in mind.
- Training programs and resources are available to help individuals without technical backgrounds learn about and engage with AI.
- The democratization of AI empowers individuals from various disciplines to utilize AI for their specific needs.
5. AI is solely focused on humanoid robots
Movies and popular media often depict AI as humanoid robots, leading to the misconception that AI is only about physical entities. In reality, AI encompasses a wide range of applications beyond robotics.
- AI is used in healthcare to improve diagnoses and treatment plans.
- AI is utilized in recommendation systems for personalized suggestions, such as in streaming platforms or online shopping.
- AI powers natural language processing systems, like virtual assistants, that assist with everyday tasks.

AI vs. Humans: Comparison of Capabilities and Limitations
As artificial intelligence (AI) continues to advance, it is worth exploring how it compares to human capabilities and the limitations it still faces. This article presents 10 tables highlighting various aspects of AI and human abilities, shedding light on the ongoing debate about the potential of AI and its impact on society.
Table 1: Speed and Efficiency
When it comes to processing power and speed, AI outshines humans. While humans are limited by their biological capabilities, AI can perform millions of calculations in mere seconds, enabling rapid data analysis and decision-making.
Table 2: Creativity
Although AI algorithms have made significant strides in generating creative outputs, human creativity remains unparalleled. Humans have the capacity to create unique, original ideas that often require inspiration and emotional intelligence.
Table 3: Data Processing
AI excels in handling large volumes of structured and unstructured data, ensuring efficient data analysis. Humans, on the other hand, may struggle with vast amounts of information, often relying on AI tools for data processing and interpretation.
Table 4: Emotional Intelligence
Emotional intelligence, an essential aspect of human interaction, is challenging to replicate within AI systems. Understanding emotions, empathy, and social cues remain primarily human traits that enable nuanced communication and empathetic decision-making.
Table 5: Adaptability
Humans possess a remarkable ability to adapt to a wide range of situations, whereas AI systems are limited to the scope of their programming. Humans can learn and innovate in dynamically changing environments, giving them a competitive edge over AI.
Table 6: Error Rates
AI systems, operating on logical algorithms, usually exhibit lower error rates compared to humans, who are susceptible to cognitive biases and human errors. However, AI can still make mistakes if it encounters unanticipated scenarios or flawed input data.
Table 7: Ethical Decision-making
Ethics are deeply ingrained in human decision-making, allowing for complex moral judgments in ambiguous situations. While AI can be programmed with ethical frameworks, it struggles to deal with ethical dilemmas that require context and subjective interpretation.
Table 8: Learning Capacity
Both humans and AI systems are capable of learning, but their approaches differ. Humans possess the ability to learn autonomously, adaptively, and creatively, drawing connections from diverse experiences. AI systems require extensive training data and continuous refinement to improve their learning capacity.
Table 9: Physical Abilities
Humans possess physical dexterity and are capable of performing intricate tasks that require fine motor skills. AI systems lack physical presence and are limited to processing information, unable to replicate the wide range of physical movements humans can effortlessly execute.
Table 10: Morality and Consciousness
The realms of morality and consciousness are unique to humans. While AI can simulate behavior and decision-making based on guidelines, ethics, and data analysis, it lacks consciousness and moral reasoning, making it fundamentally different from human intelligence.
In conclusion, AI and human capabilities possess distinct strengths and weaknesses. AI proves advantageous in areas such as speed, data processing, and error rates, while human creativity, adaptability, emotional intelligence, and ethical decision-making continue to outperform current AI systems. Recognizing the synergistic potential of human-AI collaboration and understanding the boundaries of AI is vital to harnessing its capabilities effectively in a rapidly evolving world.
Frequently Asked Questions
What is the difference between AI and Machine Learning?
AI, or Artificial Intelligence, is a broad concept that refers to the development of machines or systems exhibiting intelligent behavior. On the other hand, Machine Learning is a subfield of AI that focuses on enabling machines to learn from data without being explicitly programmed. While AI encompasses various techniques, Machine Learning is a specific approach within the AI domain.
How does AI impact our daily lives?
AI has numerous applications that impact our daily lives. It can be found in voice assistants like Siri and Alexa, recommendation systems, autonomous vehicles, personalized advertisements, fraud detection systems, and much more. AI technologies are increasingly being integrated into various industries, making our lives more efficient and convenient.
What are the main benefits of using AI?
AI offers several benefits, including increased efficiency, improved accuracy, enhanced decision-making capabilities, and the ability to automate repetitive tasks. Additionally, AI can analyze large amounts of data to uncover valuable insights, detect patterns, and predict future outcomes. These advantages make AI highly sought after across industries.
What are the limitations of AI?
Despite its capabilities, AI also has certain limitations. Some common challenges include the lack of human-like common sense reasoning, the potential for bias in decision-making algorithms, data privacy concerns, and the fear of job displacement. It is crucial to address these limitations while developing and deploying AI systems to ensure their responsible and ethical use.
How does AI relate to job automation?
AI can automate various tasks and even some jobs that are repetitive or rule-based. However, it is important to note that AI does not necessarily mean complete job replacement. Instead, AI technologies often enhance human capabilities, augmenting human work rather than replacing it entirely. Many jobs will continue to require human skills like creativity, critical thinking, and interpersonal communication.
What is the difference between narrow AI and general AI?
Narrow AI, also known as weak AI, refers to AI systems designed for specific tasks or domains, such as voice recognition or image classification. In contrast, general AI, also called strong AI, aims to create machines capable of performing any intellectual task that a human can do. While narrow AI is prevalent today, general AI remains a goal for future development.
Is AI dangerous or could it become a threat to humanity?
The potential dangers of AI largely depend on its development and use. While there are concerns about AI’s impact on employment, privacy, and security, the idea of AI becoming an existential threat to humanity, as portrayed in popular culture, is speculative. Responsible development, regulation, and ethical considerations are crucial in ensuring the safe and beneficial deployment of AI.
Are there any ethical issues associated with AI?
Yes, the ethical implications of AI are significant. Some ethical concerns include data privacy, algorithmic bias, transparency and explainability of AI systems, accountability for AI decisions, and the potential for AI to reinforce existing social inequalities. Addressing these issues is essential to ensure that AI technologies are developed and used ethically and for the benefit of all.
How does Machine Learning contribute to AI?
Machine Learning techniques enable the development of AI systems by allowing them to learn from data and improve their performance over time. Machine Learning algorithms make it possible for AI systems to automatically learn and adapt to new information without being explicitly programmed for each specific task. Machine Learning plays a vital role in the advancement of AI technologies.
What are some notable real-world applications of AI?
AI finds application in many domains. Examples of notable real-world applications include autonomous vehicles, virtual assistants, fraud detection systems, healthcare diagnosis and treatment planning, recommendation systems in e-commerce platforms, smart home devices, and intelligent personalization of online services. AI is continuously evolving and expanding its reach across various industries.