Acquisition in AI
Artificial Intelligence (AI) has become a rapidly growing field, with various companies striving to acquire new technologies and startups to stay ahead of the competition. These acquisitions not only enable companies to expand their capabilities but also foster innovation and drive advancements in the AI industry. In this article, we will explore the importance of acquisition in AI and how it shapes the landscape of this transformative technology.
Key Takeaways:
- Acquisitions in AI are crucial for companies’ growth and competitiveness.
- They facilitate the expansion of capabilities and access to new technologies.
- Acquisitions foster innovation and drive advancements in the AI industry.
**Acquisition opportunities in AI** arise due to the rapid advancements and constant evolution in the field. Companies seek to acquire AI startups or technologies to harness their valuable intellectual property and expertise. These acquisitions enable companies to accelerate their AI initiatives and gain a strategic edge over competitors. Acquiring established AI players can also provide instant access to a talented workforce and an existing customer base, expediting the deployment of AI solutions.
*For example, Google’s acquisition of DeepMind in 2014 allowed them to leverage DeepMind’s extensive experience in deep learning and reinforcement learning, advancing their AI capabilities in several areas, including healthcare and autonomous vehicles.*
**Benefits of acquisition in AI** extend beyond technology integration. Acquiring an AI startup brings together the expertise of both organizations, fostering collaboration and knowledge-sharing among talented individuals. This synergy often leads to groundbreaking advancements and innovative solutions. Moreover, acquisitions assist in mitigating talent shortages by attracting and retaining top AI talent through attractive career development opportunities and resources.
*By acquiring the AI startup Nervana Systems, Intel enhanced its AI portfolio and established itself as a key player in the AI space, benefiting from Nervana’s deep learning technology and expert team.*
Company | Acquired by | Value | Year |
---|---|---|---|
DeepMind | $500 million | 2014 | |
Nervana Systems | Intel | $400 million | 2016 |
SwiftKey | Microsoft | $250 million | 2016 |
**Challenges in AI acquisitions** arise from the complexity of integrating acquired technologies into existing systems. Combining different AI frameworks, algorithms, and architectures can present compatibility issues and require significant effort to align and optimize performance. Additionally, integrating diverse organizational cultures and ensuring effective collaboration between teams from different backgrounds can pose challenges in achieving desired outcomes and realizing the full potential of acquired AI expertise.
*Overcoming these challenges requires careful planning, effective communication, and investment in cultural integration strategies, ensuring a smooth transition and maximizing the value of the acquisition.*
Factor | Description |
---|---|
Strategic Alignment | The acquisition aligns with the buyer’s long-term strategic goals. |
Technological Compatibility | The acquired technology can be effectively integrated with existing systems. |
Talent Retention | The acquisition aids in attracting and retaining key AI talent. |
- Looking ahead, **the pace of AI acquisitions** is expected to continue as companies strive to strengthen their position in the AI market and tap into new areas of innovation.
- **Cross-industry acquisitions in AI** will become more prevalent as companies recognize the potential of AI-powered solutions across various sectors, from healthcare and finance to transportation and manufacturing.
- The **ethics of AI acquisitions** will become an increasingly important consideration. Companies must carefully evaluate the social and ethical implications of acquiring AI technologies, including the responsible use of AI and its impact on privacy, fairness, and accountability.
Acquisition plays a vital role in shaping the AI landscape, driving innovation, and propelling the technology forward. As AI continues to advance, companies that strategically acquire AI startups and technologies will be better positioned to create groundbreaking solutions and gain a competitive edge in the rapidly evolving AI industry.
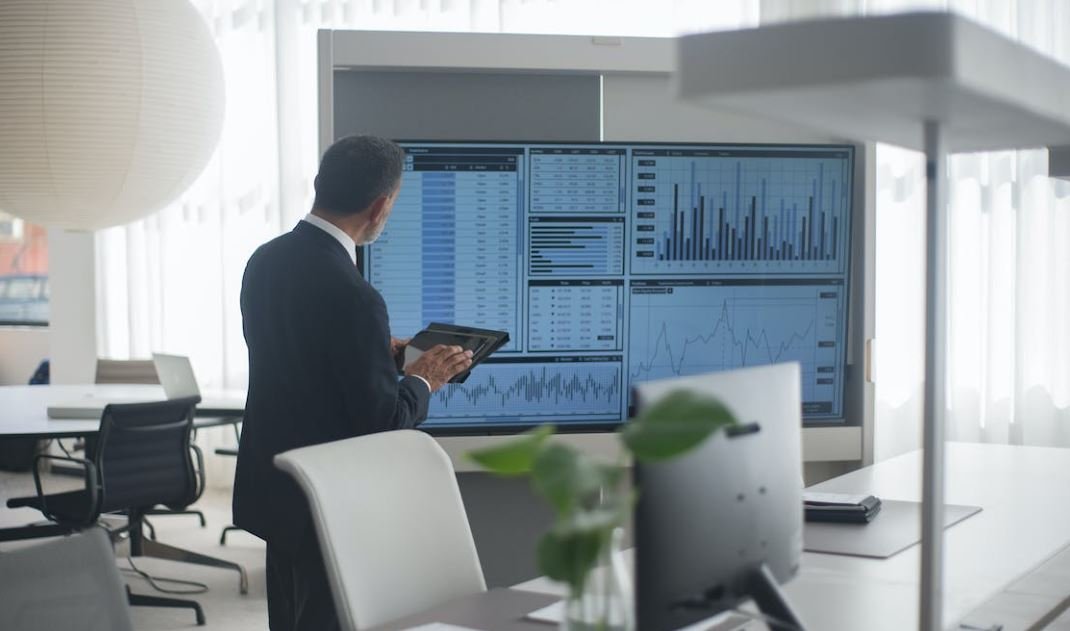
Common Misconceptions
Acquisition in AI
Acquisition in AI is a complex and often misunderstood topic, leading to several misconceptions. One common misconception is that AI acquisition is primarily focused on obtaining data. While data is indeed essential for training AI systems, acquisition in AI goes beyond just data gathering. It also involves obtaining algorithms, models, and even entire AI systems.
- Data gathering is only one aspect of AI acquisition
- Acquisition involves obtaining algorithms, models, and complete AI systems
- AI acquisition requires a variety of resources and expertise
Another misconception is that AI acquisition only happens through purchasing or buying external resources. While acquisitions can involve buying existing AI products or services, they also encompass other methods. Organizations often acquire AI capabilities through building in-house expertise or partnering with other companies.
- Acquisitions can involve purchasing AI products or services
- Organizations can acquire AI capabilities by building in-house expertise
- Partnerships can be a form of AI acquisition
There is a common belief that acquiring AI technology will instantly solve all the organization’s problems. However, this is not always the case. AI acquisition requires careful planning and consideration of business goals. Acquiring AI technology without a clear strategy and understanding of how it aligns with the organization’s objectives can lead to disappointment and ineffective implementation.
- AI acquisition must be aligned with business goals
- Acquiring AI technology requires careful planning
- Without a clear strategy, AI acquisition can be ineffective
People often believe that AI acquisition is a one-time event. In reality, it is an ongoing process. AI technology and algorithms evolve rapidly, and organizations need to continually update and improve their AI capabilities. Additionally, as business needs change, organizations may need to acquire new AI resources or adapt existing ones to stay competitive.
- AI acquisition is an ongoing process
- AI technologies and algorithms evolve rapidly
- Organizations may need to acquire new AI resources to stay competitive
A common misconception is that AI acquisition is the domain of large organizations with vast resources. While some large organizations invest heavily in AI acquisition, smaller companies and startups can also benefit from acquiring AI capabilities. The availability of cloud-based AI services, open-source AI tools, and collaboration opportunities have made AI acquisition more accessible to a wider range of organizations.
- AI acquisition is not limited to large organizations
- Smaller companies and startups can also benefit from acquiring AI capabilities
- Cloud-based AI services and open-source tools have made AI acquisition more accessible

AI Acquisitions by Google
Google is known for its continuous focus on AI development, and one way it achieves this is through strategic acquisitions. The table below showcases some notable acquisitions by Google in the field of AI.
Company | Acquisition Date | Acquisition Amount |
---|---|---|
DeepMind | January 2014 | $400 million |
AlphaGo | March 2014 | Undisclosed |
Api.ai | September 2016 | Undisclosed |
AI Applications in Healthcare
Artificial intelligence has found numerous applications in the healthcare industry, revolutionizing patient care and diagnostics. The following table provides a glimpse into some AI applications in healthcare.
Application | Description |
---|---|
Early Cancer Detection | AI algorithms can analyze medical images to identify cancerous cells at an early stage, increasing chances of successful treatment. |
Virtual Nursing Assistants | AI-powered virtual assistants can provide basic patient care guidance, reminders for medication, and monitor vital signs remotely. |
Patient Risk Assessment | Using AI, healthcare professionals can predict patient risks, allowing for proactive interventions and preventive measures. |
AI vs. Human Performance
Can AI outperform humans in various tasks? The table below compares the capabilities of AI and humans in performing different tasks.
Task | AI | Human |
---|---|---|
Image Recognition | Can analyze millions of images per second with high accuracy. | Relies on past experiences and context to identify objects. |
Chess | AI systems like Deep Blue and AlphaZero have defeated world champions. | Highly skilled players can strategize and plan moves effectively. |
Translation | AI translation models provide fast and accurate translations across multiple languages. | Human translators can capture subtle nuances and context-specific meanings. |
AI Startups and Funding Rounds
Startup companies in AI attract significant attention and investment due to their potential for disruption. The table below lists some prominent AI startups and their funding rounds.
Company | Funding Round | Funding Amount |
---|---|---|
OpenAI | Series C | $1 billion |
SenseTime | Series D | $2.5 billion |
Cortica | Series B+ | $30 million |
AI and Job Market
As AI continues to advance, it inevitably impacts the job market. The following table highlights the potential effects of AI on different job sectors.
Job Sector | Potential Impact |
---|---|
Transportation | Automated vehicles may reduce the need for human drivers, leading to job displacement. |
Customer Service | Chatbots and AI assistants may handle a significant portion of customer inquiries, impacting customer service roles. |
Healthcare | AI can enhance clinical diagnosis, but certain roles like radiologists may be affected. |
AI in Cybersecurity
With the rise of cyber threats, AI is increasingly utilized to bolster cybersecurity defenses. The following table demonstrates how AI contributes to safeguarding digital infrastructures.
Application | Description |
---|---|
Malware Detection | AI algorithms analyze patterns to identify and defend against new and evolving malware. |
Anomaly Detection | AI systems monitor network traffic to identify suspicious activities that deviate from normal patterns. |
User Behavior Analysis | AI models scrutinize user behavior to detect potential insider threats and unusual activities. |
AI and Ethical Concerns
The growing prominence of AI raises ethical concerns that require careful consideration. The table below presents some ethical considerations associated with AI development and deployment.
Concern | Description |
---|---|
Bias in Algorithms | AI algorithms can reflect biases present in training data, resulting in unfair outcomes and perpetuating inequality. |
Job Displacement | The automation of tasks through AI may lead to job losses and socioeconomic challenges for certain workers. |
Privacy and Data Protection | AI systems often rely on vast amounts of personal data, raising concerns over privacy and unauthorized access. |
AI in Financial Services
The financial services industry benefits greatly from AI’s ability to automate tasks and improve decision-making. The table below highlights AI applications in the financial sector.
Application | Description |
---|---|
Automated Trading | AI algorithms analyze vast amounts of financial data to identify profitable trading opportunities and execute trades without human intervention. |
Fraud Detection | AI systems detect patterns of fraudulent activities by analyzing transactional data in real-time, mitigating potential financial losses. |
Risk Assessment | AI models evaluate borrower’s creditworthiness, aiding in more accurate loan approval decisions and risk management. |
AI in Entertainment Industry
The entertainment industry leverages AI to enhance user experiences and create compelling content. The table below showcases various AI applications in the entertainment sector.
Application | Description |
---|---|
Recommendation Systems | AI algorithms analyze user preferences and viewing patterns to generate personalized recommendations for movies, music, and other forms of entertainment. |
Virtual Reality | AI technologies allow for realistic simulations and immersive experiences in virtual reality applications, such as gaming and cinema. |
Content Creation | AI systems generate original content, including music and artwork, based on learned patterns and user feedback, pushing creative boundaries. |
In the world of artificial intelligence, acquisition plays a vital role in harnessing innovative technologies and expertise. Furthermore, AI is not limited to one sector but has widespread applications across various industries such as healthcare, cybersecurity, finance, entertainment, and more. While AI showcases impressive capabilities that can outperform humans in certain tasks, it also raises ethical concerns, job market implications, and privacy considerations. Despite the challenges, AI continues to evolve, disrupting traditional models and shaping a future defined by augmented intelligence.
Frequently Asked Questions
Acquisition in AI
- What is acquisition in AI?
- Acquisition in AI refers to the process of obtaining or collecting data and resources to enhance the performance of artificial intelligence systems. It involves acquiring relevant datasets, selecting appropriate algorithms, and gathering computational resources for training and development.
- Why is acquisition important in AI?
- Acquisition is crucial in AI as it directly impacts the quality and effectiveness of AI systems. By acquiring diverse and high-quality datasets, advanced algorithms, and computational resources, AI systems can learn and improve their capabilities, leading to enhanced performance in various domains like image recognition, natural language processing, and decision-making.
- How is data acquisition done in AI?
- Data acquisition in AI involves various methods such as web scraping, data labeling, data purchasing, data collection from IoT devices, and collaboration with other organizations or individuals to obtain relevant datasets. It may also include cleaning and preprocessing the acquired data to ensure its suitability for training AI models.
- What are the challenges in data acquisition for AI?
- Data acquisition for AI presents several challenges including data privacy concerns, gathering labeled or annotated data, dealing with biased or unbalanced datasets, ensuring data quality and trustworthiness, and complying with legal and ethical considerations. These challenges require careful planning and implementation to ensure the integrity and reliability of the acquired data.
- How can organizations acquire computational resources for AI?
- Organizations can acquire computational resources for AI through various means such as investing in high-performance computing (HPC) infrastructure, utilizing cloud computing services, leveraging specialized hardware like graphics processing units (GPUs) or tensor processing units (TPUs), and collaborating with research institutions or technology providers that offer AI-specific resources.
- What role does algorithm acquisition play in AI?
- Algorithm acquisition in AI involves selecting and obtaining suitable algorithms to train and deploy AI models. It is essential to choose algorithms that are appropriate for the specific task or problem at hand, considering factors such as accuracy, efficiency, scalability, and interpretability. Acquiring state-of-the-art algorithms can greatly impact the performance and capabilities of an AI system.
- How can AI acquisition be influenced by biases?
- AI acquisition can be influenced by biases when the acquired datasets, algorithms, or resources are based on biased or unrepresentative sources. Biases can result in unfair or discriminatory outcomes, reinforcing existing inequalities. It is important to address and mitigate biases during the acquisition process by considering diverse data sources, conducting bias assessments, and implementing fairness measures in AI systems.
- Can acquisition in AI result in ethical concerns?
- Yes, acquisition in AI can lead to ethical concerns. For example, acquiring personal data without informed consent or violating privacy rights can raise ethical issues. Additionally, the acquisition of sensitive information or the misuse of acquired resources can also pose ethical challenges. It is crucial for organizations to adhere to ethical guidelines and regulations when acquiring data and resources for AI.
- What is continuous acquisition in AI?
- Continuous acquisition in AI refers to the ongoing process of acquiring and updating data, algorithms, and resources to keep AI systems up-to-date and relevant. As technology advances, new data becomes available, and improved algorithms are developed, continuous acquisition ensures that AI systems can adapt and improve their performance over time.
- How can organizations ensure the security of acquired AI resources?
- To ensure the security of acquired AI resources, organizations should implement robust security measures such as encryption, access controls, vulnerability assessments, and regular audits. They should also stay updated with the latest security practices and collaborate with cybersecurity experts to mitigate risks and protect acquired AI resources from unauthorized access or malicious activities.